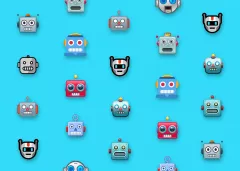
The false positive problem of automatic bot detection in social science research
A study of Botometer's diagnostic ability over time
The identification of bots is an important and complicated task. The bot classifier Botometer was successfully introduced as a way to estimate the number of bots in a given list of accounts and has been frequently used in academic publications.
Given its relevance for academic research, and our understanding of the presence of automated accounts in any given Twitter discourse, Adrian Rauchfleisch and Jonas Kaiser studied Botometer's diagnostic ability over time. To do so, Rauchfleisch and Kaiser collected the Botometer scores for five datasets (three verified as bots, two verified as human; n=4,134) in two languages (English/German) over three months.
Rauchfleisch and Kaiser show that the Botometer scores are imprecise when it comes to estimating bots, especially in a different language. They further show in an analysis of Botometer scores over time that Botometer's thresholds, even when used very conservatively, are prone to variance, which, in turn, will lead to false negatives (i.e., bots being classified as humans) and false positives (i.e., humans being classified as bots).
This has immediate consequences for academic research as most studies using the tool will unknowingly count a high number of human users as bots and vice versa. Rauchfleisch and Kaiser conclude the study with a discussion about how computational social scientists should evaluate machine learning systems that are developed to identify bots.
You might also like
- communityThe apocalypse that wasn’t