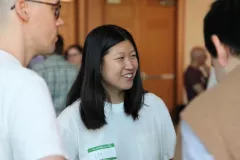
Get to Know Berkman Klein Fellow Lily Hu
a spotlight on one of our 2018-2019 BKC Fellows
by Nikhil Dharmaraj and Julia Pan
Why Computer Scientists Need Philosophers, According to a Mathematician
Lily Hu is a 3rd year PhD candidate in Applied Mathematics at Harvard University, where she studies algorithmic fairness with special interest in its interaction with various philosophical notions of justice. Currently, she is an intern at Microsoft Research New York City and a member of the Mechanism Design for Social Good research group (co-founded by Berkman affiliate Rediet Abebe). She is also passionate about education equity; she has taught subjects such as physics, biology, chemistry, English, and Spanish History/Geography in San Francisco, Cambridge, and Madrid.
Read more stories from our Interns and Fellows!
Note: Lily Hu was Julia’s high school calculus tutor. At that time, neither of them knew they were interested in machine learning ethics. The Berkman Klein Center has unwittingly brought them together again, where Julia and Nikhil were assigned to interview Lily, three years later.
How are algorithms distributing power between people? What kind of questions are they enabling us to ask, what kind of questions are they enabling us to solve, and not only that, but what kind of questions are they preventing us from answering?
JULIA: Can you elaborate on the work you're doing at the Berkman Klein Center — an overview of the project, where you’re at right now, and where you hope to be at the end of the 1-year fellowship?
I work in algorithmic fairness; in particular, I’m interested in thinking about algorithmic systems as explicitly resource distribution mechanisms. I’m not interested in necessarily how the particulars of the sorting happens; I’m interested in the final outcomes that are issued, and I am interested in the distributional outcomes that are deemed to be appropriate or inappropriate under our various fairness notions. How are algorithms distributing power between people? What kind of questions are they enabling us to ask, what kind of questions are they enabling us to solve, and not only that, but what kind of questions are they preventing us from answering? That’s kind of my big research agenda.
What I am focusing on at the Berkman Klein Center in particular is how those algorithms actualize particular political philosophies. So, for example, Robert Nozick is a well-known libertarian philosopher, and he believed very strongly in the idea that some sorts of procedures are fair, just by virtue of their being agreed upon by consenting free individuals. That’s basically a tenet of classical libertarianism. Right now, there’s a big tendency within the computer science community to think of consent as a big aspect of what makes something fair — “Oh, you consented to having your privacy taken away from you when you logged on to Facebook.” So I ask, what are the actual political philosophies embedded in each of these algorithms when we say that they’re fair? A lot of that is going to the background, getting pushed aside, because we’re just thinking about how to sort more efficiently.
JULIA: When do discussions of algorithmic fairness have to include political philosophy?
Well, this is what I’ve been trying to say to computer scientists for years: if you want to play chess, you have to learn the rules! There’s no such thing as just a technical solution. If computer scientists are going to get into this field, they need to familiarize themselves with exactly what they’re getting their hands involved in and respect the long tradition (we’re talking like older than Plato) of conversations in what we owe each other in our makings of a society. For me, is the most important question of our time. What do we owe each other as we design our institutions, as we try to live together in a society? What are the basic things that every person ought to have? And that’s not something that you just get from intuition; it’s something that really needs to be studied.
I’ve been trying to say to computer scientists for years; if you want to play chess, you have to learn the rules! There’s no such thing as just a technical solution.
NIKHIL: Why do you feel that BKC is the best incubator for this kind of research, and also, what does your work for this kind of research look like? How are you planning to investigate these questions specifically?
Berkman Klein is one of the institutions that has been doing this work the longest; it has recognized the important intersection between Internet/technology and society. It brings in a perspective not embedded in an engineering program or technology program, which I think is super helpful. There is a particular spin on things when you’re working from a tech industry, from within a school of engineering. But the work that I’m doing fundamentally — I’d honestly get more help from talking to anthropologists and legal scholars and sociologists than I would from talking to another machine-learning person. And the fact that I happen to be a grad student at Harvard, and the fact that I have a perennial existential crisis about whether I go to law school...just two more things that say, “Be a part of Berkman Klein.” Those are the perspectives — the community — the people who have been much more critical thinkers on this topic for the longest time and continue to be leaders in it. And just having that exposure to the community is so important.
What I do daily? It’s not sexy. I read a bunch of philosophy, which is honestly great because it’s one of my favorite things to do anyways. Recently, I’ve been really interested in this sub-literature on causality and counterfactual fairness. So machine learning people writing about what it means to make something causally or counterfactually fair.
Sometimes I’m angry. I’ll call up some friends. I actually did yesterday — actually, in the middle of the day, I was like I need to respond to this immediately. I wrote an email to a friend of mine, I called him, and we met up. And I was like, “OK, let’s talk about why this is wrong.” So it’s literally just that! Read a fairness paper, read a bunch of philosophy, talk to people I know, and then just think about what is going on here. What is the technical move that is making this possible? And then what is the justification for the algorithmic move? It’s a lot of reading, a lot of talking to people — both of those things I like. But I’m not out there like a data warrior, getting people to hand me their tax returns or something.
JULIA: We love your work, we’re both also really passionate about algorithmic fairness. How did you specifically get into the intersection of AI & Ethics, especially since a lot of your undergraduate research seemed focus on math and evolutionary biology?
It’s just kind of luck to be honest. I came to Harvard for graduate school, knowing that I’d have a lot more opportunities to do the interdisciplinary work I wanted to do. I was two months into my first year, and then Trump became president. The day after that, I was sitting in my stochastic processes class, and we were doing random matrices. I remember this so starkly — I was just like, what is the point? I’m sitting here doing random matrices and I’ll probably never use them. And hate crimes have ticked up, what? 180%? Like what are we doing? At every single moment, we are making a decision — to be a part of something or to not be a part of it. And I realized in that moment, that there was really no justification for me to continue grad school, at all. So, I was having this conversation with my advisor, and she introduced me to this relatively new subdomain that people had started working in about where they were thinking about biases in machine learning. And I realized I want to work on that. I think I just got incredibly lucky — or the world got incredibly unlucky — by the election in 2016, which timed perfectly with my own sense of commitment and wanting to stand for something. At that moment, the field of algorithmic fairness hit an inflection point — where what they were thinking about was not only under the purview of experts but also of popular concern and input. It opened up the opportunities for people down here, on the lowest rungs of the academic ladder, to make an impact.
NIKHIL: Where do you see yourself and your work in 10 years?
I hope to be in academia, I guess that’s a logistical answer to that. The reason I would like to be in academia is because I don’t foresee any of the things I’m interested in, or the problems I’m trying to solve, or the kinds of research I want to take part in, or the colleagues I want to talk to — I don’t think any of that is aligned with what a corporation does. I think fairness is “in” for companies like Google, but in the liability-avoidance sense. I think I would be really dissatisfied with that.
And that’s also one of the reasons I wanted to come to BKC — this is the intellectual, academic environment I want to be in. I don’t want to be pigeon-holed into any traditional department, because I think the kind of work that I’m doing will require me to always stay open. But I do hope to continue to be at a higher-level institution of learning.
NIKHIL: So, when you’re not working on these fascinating academic questions, what do you do in your free time? What are your hobbies?
I audit algorithms in my free time...I just told you what I do in my free time! Just kidding. OK, I love cooking, and I love baking. Just made the best granola...ever (it’s from Eleven Madison Park). I run a lot. I play basketball. I read a lot of arts journalism - music journalism.
JULIA: What is one question we didn’t ask you?
Hmm...you didn’t ask me why I think why algorithms are important NOW.
JULIA: And what’s your answer?
I think there’s a lot of mystique around algorithms — there are many reasons why they have generated so much buzz. I think they’ve captured not only the imagination of Silicon Valley Bros, but they also capture the imagination of a lot of regular people. There’s something strange going on — everyone hates big data, hates their privacy being encroached upon. But they simultaneously also are addicted to the mythical powers of AI. The fact that we can distill order out of chaos — that’s the story of Big Data.
But I think that the application of machine learning to social sciences is very dangerous — machine learning breaks some sort of fundamental social and political bond by getting all this data, and treating us simultaneously as just these atoms (the sums of our features), but also eviscerating the individual at the same time, because everyone just becomes the average. I just become an Asian woman who is 24. I simultaneously become nothing but my features, which makes me incredibly individualized. But also under Big Data, I become nothing but those features, which makes me very generic. The fact that machine learning can slip in and out of these two opposite scopes of analysis makes it very powerful tool of both surveillance and disciplinary power. It’s why algorithms need to be studied by all types of people.
Read more about our Open 2019 - '20 Fellows Call for applications!
Interviewers
Nikhil Dharmaraj
Nikhil is a rising senior at The Harker School (San Jose, CA) fascinated by the academic space lying at the intersection of technology and the humanities. At Berkman, he is an intern at the metaLAB working on the Laughing Room and Moral Labyrinth projects. In his free-time, he enjoys reading obscure books, learning new instruments, and playing with his fluffy, adorable dog.
Julia Pan
Julia is a Boston native and rising senior at the University of Pennsylvania studying Cognitive Science, Economics, and Innovation & Technology Policy. At Berkman, she is on the Ethics and Governance of AI project. You can find her singing, taking photos, obsessing over design and fonts, or rambling about behavioral economics and civic technology in her free time.