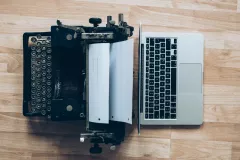
Accounting for AI — a Comparison of Methods
A few years ago, Finale Doshi-Velez and Mason Kortz – and a squad of collaborators – unpacked explanation as one way to hold AI systems accountable. Many frameworks for AI regulation and best practices have since emerged.
In this conversation, Finale and Mason returned to the question of accountability and regulation for AI: What are the options for regulating AI systems in real-world settings? What are the advantages of ex-ante and ex-post monitoring? What roles should different methods of accountability play? How do we strategically use each one?
They reflected on how their views have evolved and considered some ways forward.
Recommended Resources:
- Finale Doshi-Velez, Mason Kortz, et al., Accountability of AI Under the Law: The Role of Explanation
- Amba Kak, Rashida Richardson, Artificial Intelligence Policies Must Focus on Impact and Accountability
- MIT/Assembly, AI Blindspot
- Been Kim, Finale Doshi-Velez, Machine Learning Techniques for Accountability
(Header image by Glenn Carstens-Peters on Unsplash.)
You might also like
- communityThe apocalypse that wasn’t